The Future of AI: An Overview
Artificial Intelligence is steadily becoming the backbone of technological progress in the digital era, with its advancements underpinned by a variety of fields, each specializing in enhancing machine intelligence in distinctive ways.
Among these are Machine Learning (ML), Reinforcement Learning (RL), Data Mastery, Natural Language Processing and Robotics, which form the triad of AI innovation.
This article delves into the roles these disciplines play in crafting the AI-driven future, highlighting their individual significance in reshaping the technology landscape.
1.Machine Learning: The Engine Behind AI Evolution
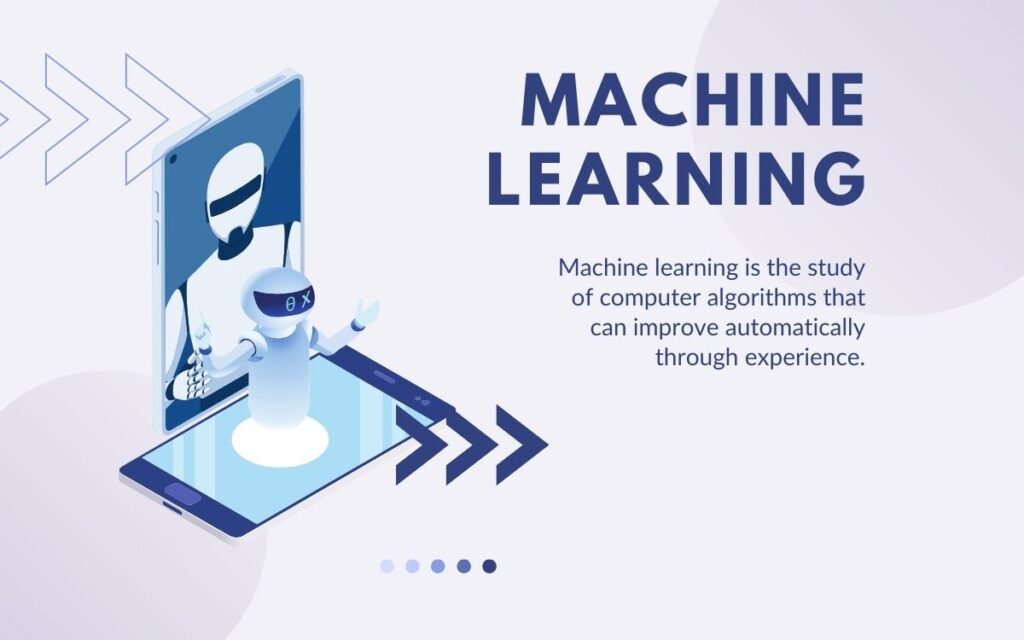
Machine Learning (ML) stands at the nucleus of AI progression, enabling systems to absorb knowledge from data autonomously, without the need for manual programming.
By training algorithms to identify patterns and make data-driven decisions, ML becomes an indispensable tool.
Key Elements of Machine Learning:
- Supervised and Unsupervised Models: Learning occurs either with labeled datasets (supervised) or through the discovery of hidden patterns in unstructured data (unsupervised).
- Use Cases: Fraud detection, recommendation engines, autonomous driving technologies.
- Algorithms: Decision trees, neural networks, support vector machines.
ML’s prominence in the AI ecosystem stems from its role in automating functions, forecasting outcomes, and optimizing decision-making processes across sectors.
Whether it’s revolutionizing healthcare diagnostics or powering autonomous vehicles, ML will continue to steer the AI ship into uncharted territories as datasets expand.
2.Reinforcement Learning: Enhancing Dynamic Decision-Making
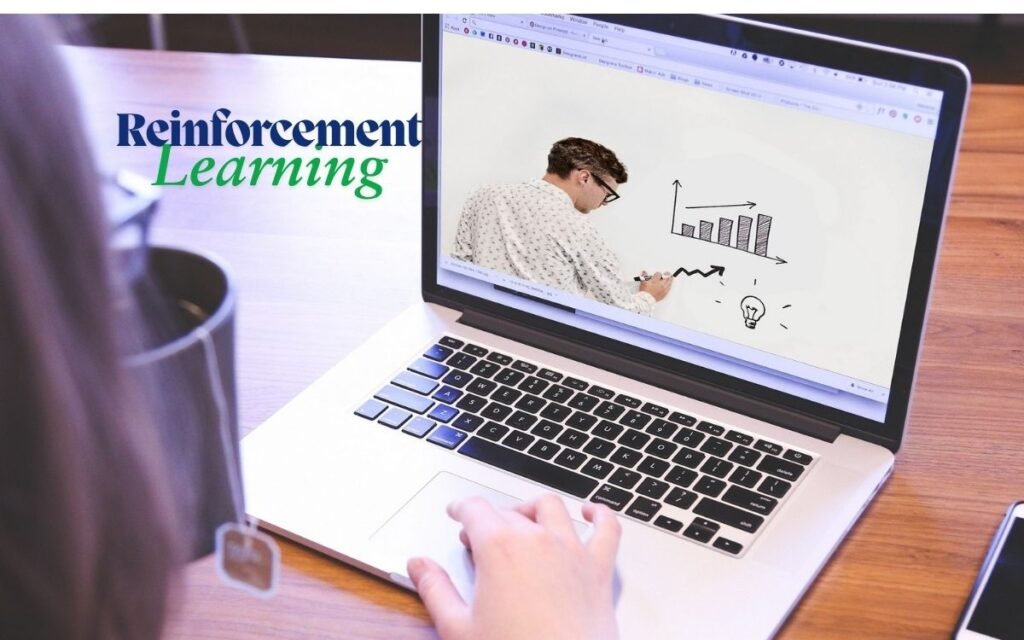
Reinforcement Learning (RL), a subset of ML, focuses on teaching agents to make sequential decisions by interacting with their environment.
These agents refine their strategies by evaluating outcomes through rewards and penalties.
Core Principles of Reinforcement Learning:
- Explorative Learning via Trial and Error: Agents experiment with various actions, learning to improve based on positive or negative reinforcements.
- Applications: Robotics, game AI, self-governing systems, real-time analytics.
- Key Algorithms: Q-learning, Deep Q-Networks (DQNs), Policy Gradient techniques.
RL is integral to systems where real-time decision-making is paramount, particularly in autonomous environments.
Its self-adaptive capabilities will fuel future innovations in robotics, intelligent city management, and automated trading platforms, ensuring these systems evolve and optimize continuously.
3.Data Mastery: The Bedrock of Smart AI Systems
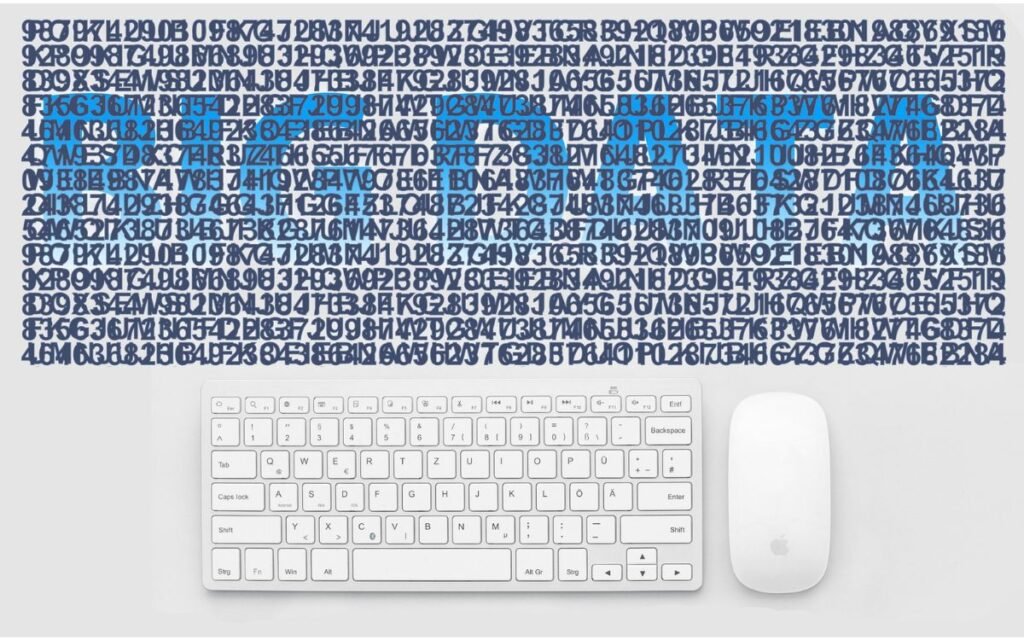
Data Mastery, often intertwined with Big Data Analytics and Data Science, is the process of sifting through vast quantities of data to derive actionable insights.
AI systems rely on this data-driven intelligence to fine-tune their decision-making processes.
Key Aspects of Data Mastery:
- Data Mining & Predictive Analysis: Extracts valuable patterns from colossal datasets.
- Applications: Healthcare analytics, economic forecasting, targeted advertising.
- Technological Foundations: Hadoop, Apache Spark, SQL, and NoSQL architectures.
As the digital world churns out an ever-growing volume of data, the importance of Data Learning intensifies.
It allows AI systems to transition from raw information to meaningful insights, helping industries like healthcare, finance, and commerce to make data-informed decisions with unparalleled accuracy.
4.Natural Language Processing: Humanizing AI Dialogue
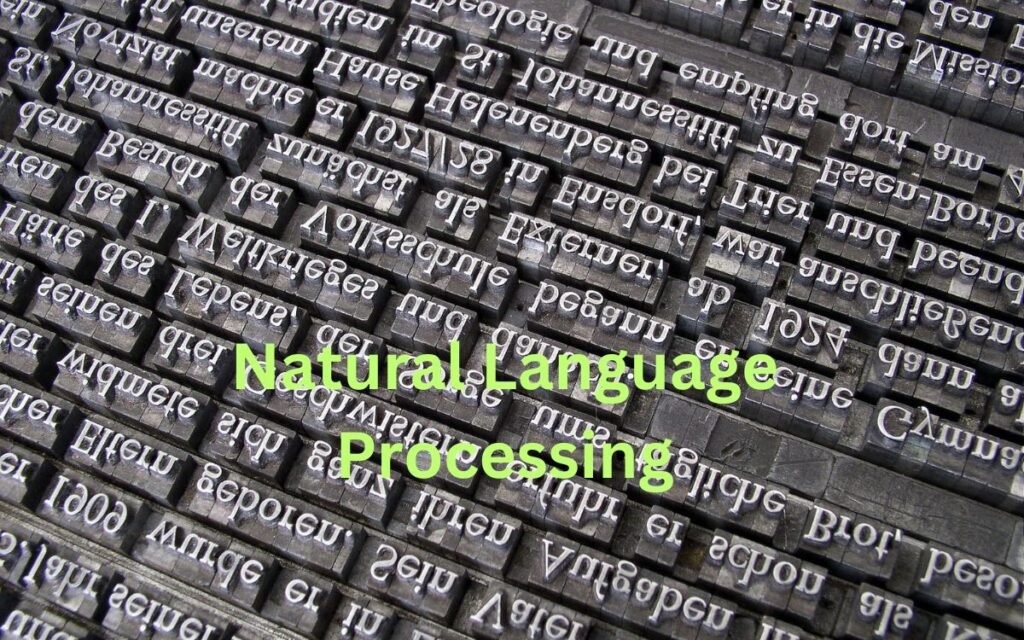
Natural Language Processing (NLP) enables machines to comprehend, interpret, and respond to human languages, combining computational linguistics with ML.
This synergy fosters a more intuitive interaction between AI systems and users.
Key Aspects of NLP:
- Speech Recognition & Text Synthesis: Converts spoken words to text and generates human-like responses.
- Applications: Virtual assistants (e.g., Siri, Alexa), customer service chatbots, sentiment analysis, language translation.
- Technologies Involved: Transformers (BERT, GPT), Recurrent Neural Networks (RNNs), word embeddings.
NLP will remain pivotal as AI interfaces increasingly embed themselves in everyday life, ensuring seamless, human-like communication between users and machines.
5.Robotics: The New Era
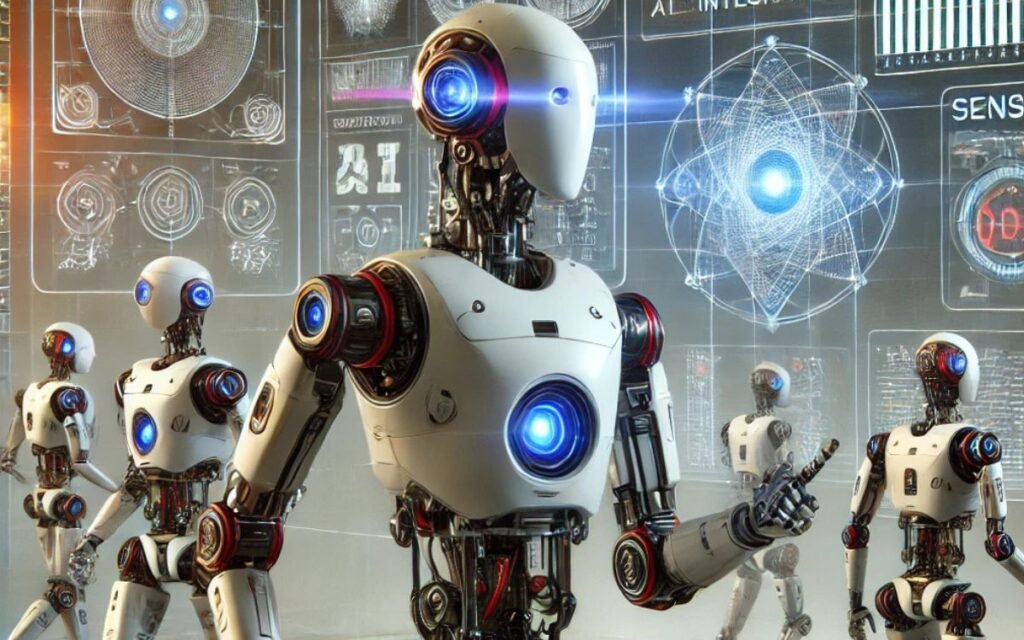
Robotics is a rapidly advancing field that combines engineering, artificial intelligence, and automation to design machines capable of performing tasks autonomously or with human guidance.
Key aspects of robotics include motion control, where robots are programmed to move with precision; sensors and perception, enabling robots to interpret their environment; and machine learning, which allows robots to improve performance through data-driven learning.
Applications span industries from manufacturing and healthcare to exploration and service robots, making robotics essential for automating complex, real-world tasks.
Key Aspects of Robotics:
- Autonomy: The ability for robots to perform tasks with minimal human intervention, using pre-programmed instructions or learning algorithms.
- Motion Control: Precise movement and manipulation of objects, which is achieved through motors, actuators, and complex control systems.
- Sensors and Perception: Robots are equipped with sensors (such as cameras, infrared, and touch sensors) to perceive their surroundings and make decisions based on environmental data.
- Artificial Intelligence (AI) Integration: AI allows robots to learn, adapt, and improve their performance over time by analyzing data and optimizing actions.
- Human-Robot Interaction (HRI): The study and design of systems that allow for effective communication and collaboration between robots and humans, often through speech or gesture recognition.
- Mechanical Design: The physical structure of robots, including arms, legs, and other components, designed for stability, strength, and the specific tasks they perform.
- Programming and Control Systems: The software that drives robotic behavior, controlling everything from basic movements to complex decision-making processes.
- Power and Energy Management: Efficient energy systems that ensure long operation times, which can include batteries, solar power, or hybrid energy systems.
How These Disciplines Shape the AI Landscape
- Interdisciplinary Integration
ML, RL, Data Learning, and NLP will each shape different sectors, from the automation of routine tasks to the optimization of intricate decision-making systems.
While ML propels automation forward, RL fine-tunes decision processes, Data Learning ensures predictive precision, and NLP enhances human-AI interaction.
- Advanced Applications
We will witness AI’s further evolution through autonomous systems, advanced real-time analytics, and interactive AI platforms.
Reinforcement Learning will power robotics and self-driving systems, while NLP will refine AI-driven customer service, content creation, and translation tools, bringing these systems closer to human-like interaction.
- Ethics and Responsibility in AI
As AI systems grow more sophisticated, ethical considerations surrounding ML, RL, and Data Learning must be carefully addressed. Ensuring fairness, transparency, and accountability in AI models will become increasingly important.
Training AI on diverse datasets and monitoring decision-making will be essential to ensure equitable outcomes across applications.
For more information: https://builtin.com/artificial-intelligence/artificial-intelligence-future
FAQ: The Future of AI
Q.How does Machine Learning differ from Reinforcement Learning?
A.Machine Learning (ML) focuses on recognizing data patterns, either supervised or unsupervised. Reinforcement Learning (RL), however, centers on agents learning through environmental interaction, refining strategies via rewards and penalties.
Q.Why is Data Learning significant for AI?
A.Data Learning empowers AI systems to sift through enormous datasets, uncovering patterns and insights that refine decision-making processes. This is essential for enhancing predictive accuracy in businesses and optimizing operations across industries.
Q.What are the practical uses of NLP?
A.NLP has diverse applications, from powering virtual assistants like Siri and Alexa to enabling customer service chatbots, conducting sentiment analysis on social media, and facilitating language translation. It allows AI to engage in natural language conversations with humans.
Q.How does Reinforcement Learning enhance autonomous systems?
A.RL is ideal for autonomous systems such as robots and self-driving vehicles because it enables real-time decision-making based on environmental feedback. By learning from these interactions, RL optimizes the behavior of these systems to achieve desired goals more efficiently.
For more information: https://toptenplus.in/digital-india-corporation/